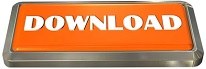

Therefore, the optimal number of remaining attention modules to be added is evaluated in this study. But the excessive addition of residual attention modules is likely to make the neural network too deep, resulting in a gradient diffusion or explosion effect 6, 7, and finally making the model classification effect not rise but fall. It is worth mentioning that residual attention mechanism improves the classification accuracy by stacking residual attention modules. Thus, more valid information can be obtained on a single image, making CAD less dependent on large amounts of data during its application in primary hospitals. At present, the problems of a small number of gastroscopic screening cases and insufficient high-level experts in China’s grass-roots hospitals have put forward new challenges to the promotion and use of CAD in grass-roots hospitals.Residual attention mechanism is to embed the residual attention module into the model, and the residual structure, the soft mask branch, in the module strengthens the ability of the model to extract the good features of the picture 5. Although the strategy of enhancing neural networks with attention mechanisms has improved the accuracy of CAD technology in the screening of gastroscopic lesion pictures, the effect of these optimization strategies in real application scenarios still needs to be further verified.Įstablishing a tumor screening prevention and control system in primary medical institutions is an effective strategy to achieve early diagnosis and treatment of tumors. used a NonLocal mechanism to further enhance the neural network, which can improve the classification accuracy of benign and malignant data of gastric ulcer lesions to 96.79% 4. proposed that the F1 score of gastric cancer detection can reach 89.95% through the combination of feature fusion module and channel attention mechanism 3. The application approach of CAD in gastroscopic image-aided recognition is mainly based on the method of image recognition, that is, the initial network is trained by first extracting the relevant features of the image, such as color, texture, shape and spatial relationship, and then using the trained network to classify the lesion type of the corresponding lesion area. In recent years, computer-assisted diagnosis (CAD) in medical imaging has received widespread attention for its important value in clinically assisted diagnosis and training 2.
Cross entropy loss function how to#
At present, how to improve the ability and level of early screening of gastrointestinal cancer in grass-roots hospitals is an urgent problem to be solved. Gastrointestinal endoscopic screening in high-risk populations is still an effective means of early diagnosis and early treatment of gastric cancer. In China, gastric cancer is second only to lung cancer, ranking second in the incidence of cancer 1. Gastric cancer is a major malignant tumor that threatens human health and is one of the six most common cancers in the world. The residual attention mechanism can effectively improve the classification effect of Xception convolutional neural network on benign and malignant lesions of gastric ulcer on common digestive endoscopic images.
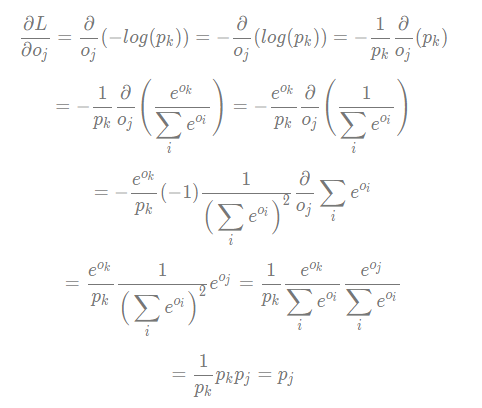
The pretreatment of digestive endoscopy can remove the interference information on the digestive endoscopic image data extracted from the database, which is beneficial to the training of the model.

The superposition of residual attention modules can effectively improve the feature learning ability of the model.
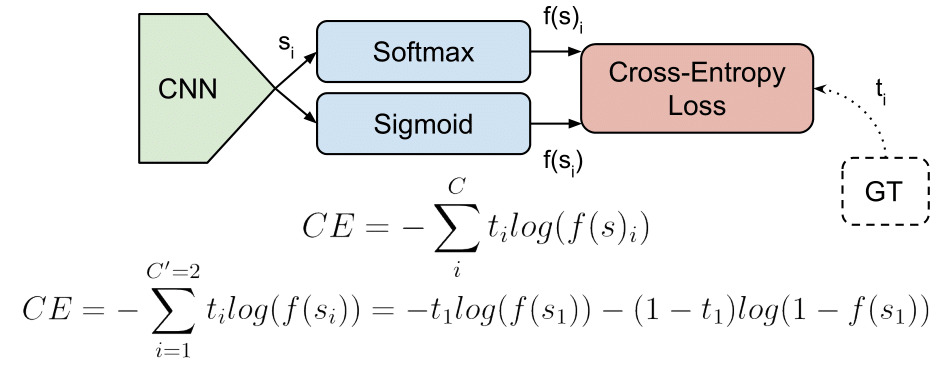
The accuracy, F1 score, sensitivity, specificity and precision of the Xception model improved by the residual attention module for the diagnosis of benign and malignant gastric ulcer lesions were 81.411%, 81.815%, 83.751%, 76.827% and 80.111%, respectively. The model is based on convolutional neural network for automatic classification and diagnosis of benign and malignant gastric ulcer lesions in small number of digestive endoscopy images. The algorithm model is implemented by Pytorch, and the preprocessed image is used as input data. For the problems of uneven illumination and low resolution of endoscopic images, the original image is preprocessed by Sobel operator, etc. To explore the application value of convolutional neural network combined with residual attention mechanism and Xception model for automatic classification of benign and malignant gastric ulcer lesions in common digestive endoscopy images under the condition of insufficient data.
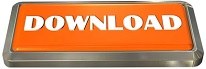